Attribution has become complicated following Apple’s privacy changes. It’s been years since their introduction, yet mobile advertisers are still looking for ways to stabilize the performance.
They are left with two main options for attribution at scale on iOS: Apple’s SKAdNetwork, also known as SKAN, and probabilistic attribution.
The switch followed by the introduction of SKAN has significantly complicated attribution, made it more challenging to scale users on iOS, and diminished reliability for advertisers. It’s almost killed attribution for rewarded ads, which users enjoy for the value they provide compared to regular mobile ads.
Luckily, probabilistic attribution now covers the areas where SKAN has been lacking for advertisers, such as data detail and instant processing – and SKAN has also improved significantly since it was first introduced.
What Is Probabilistic Attribution?
Probabilistic attribution is a method used to track and understand how users interact with ads that allows to assign credit for desired marketing outcomes. It uses probability to figure out how much each touchpoint, like an impression or an ad click, contributes to the goal, such as an installation.
And here’s how it works:
- It assigns a chance of conversion to each touchpoint based on various data.
- It matches clicks to actions (for example, installs) using details such as IP addresses and user agents (information about the device and browser).
- The method assumes the user’s IP address stays the same between clicking an ad and installing the app. In mobile game user acquisition, the user’s IP in fact rarely changes between a click and an install. In most cases, these two events are just minutes apart – the user would click and then install within less than five minutes, while still holding the same identifiers.
- Other details, such as device language and model, help confirm these matches.
Probabilistic attribution is very accurate within a typical 24-hour period after a user has interacted with an ad, meaning that advertisers can optimize and analyze their campaigns based on reliable real-time data.
Which is Better: Probabilistic Attribution or SKAN?
When comparing SKAN and probabilistic attribution, each has its strengths and weaknesses.
SKAN is privacy-centric to protect user identities, which is its main strength. Though great for the user, this limits the information available to advertisers and makes user behavior analysis and campaign optimization challenging.
iOS users, since the privacy updates, now need to share their IDFA – and currently, around three in four users opt out of doing so. This leaves advertisers unable to attribute users deterministically at scale.
Probabilistic attribution doesn’t breach Apple’s restrictions in that it doesn’t uniquely identify users but uses aggregated data points to infer user actions. This method instead gives advertisers access to more detailed data and better campaign measurement and optimization opportunities.
Advertisers that moved from deterministic attribution to solely using SKAN can no longer expect a granular breakdown of their revenue per paid media channel. This is because SKAN only permits up to three post-install event postbacks – only a subset of the true number of conversion events.
Probabilistic attribution, while not 100 percent accurate, allows advertisers to measure their campaigns more precisely, with no restrictions on post-install performance analysis.
SKAN also struggles with recognizing re-installs. Due to anonymized data, it’s impossible to differentiate new installs from re-installs. Probabilistic attribution, however, can compare the new install data with previously recorded data and determine the likelihood of re-installs. It does so by using the same combination of IP addresses, user agents, and other nonpersonal data points.
Advertisers working with SKAN have also reported delays in receiving attributed data. The latest version of the network, SKAN 4, has improved significantly from its predecessor, introducing three conversion windows (0 to 2 days, 3 to 7 days, and 8 to 35 days). However, even the first postback is delayed by at least 24 hours.
The probabilistic method, however, gives advertisers instant access to data.
This method does have one drawback. It tends to be more computationally intensive and can be harder to grasp for those not familiar with statistics or probability theory.
How Can Advertisers Use Probabilistic Attribution with Rewarded Ads?
Rewarded ads and probabilistic attribution have become inherently linked.
That’s because rewarding users for specific actions within apps is only feasible with probabilistic attribution.
Granular user-level data enables advertisers to track and reward individual user actions, a feature that SKAN’s anonymization can’t offer. Because of SKAN’s focus on anonymity, advertisers can only see which campaign has led to an action, not which user performed it.
Even if user anonymity wasn’t an issue for rewarded advertising, the latency of SKAN attribution would still not allow for real–time reward distribution. While it’s true that the accuracy of probabilistic attribution drops after 24 hours, most installs – around 95 percent – happen within this 24-hour window, ensuring high accuracy.
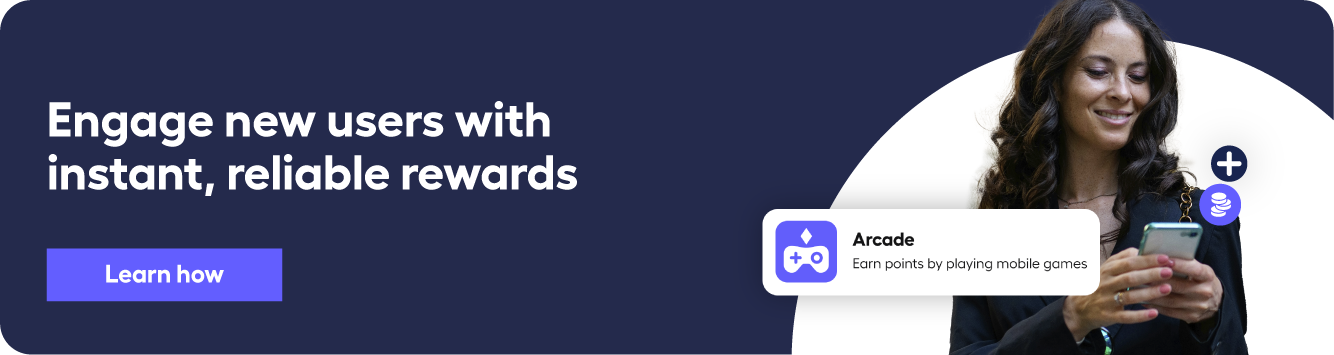
Attribution for rewarded ads on iOS is currently only possible with the probabilistic method or using a combination of SKAN and probabilistic attribution. This has been the most optimal solution – especially, for mobile advertisers with apps live on iOS or wanting to scale users on iOS.
As rewarded advertising grows in popularity and takes a larger share of user acquisition budgets, we expect probabilistic attribution to become more important.
How Advertisers Can Pivot Back to Reliable Attribution
Probabilistic attribution models – once a secondary solution for advertisers – is now becoming their primary choice since privacy changes hit the mobile advertising industry.
Its complexities have created uncertainty within circles of unprepared UA marketers, some of which still stand today. However, probabilistic attribution and SKAN have grown to be solutions that allow mobile advertisers to reliably and accurately track and attribute user actions, enabling a better understanding of user behavior.
Probabilistic attribution, or even better, a combination of both probabilistic and SKAN, is the key to receiving a quality of attribution that is close to what it once was.